Machine Learning Malware Analysis: Transforming IT Services
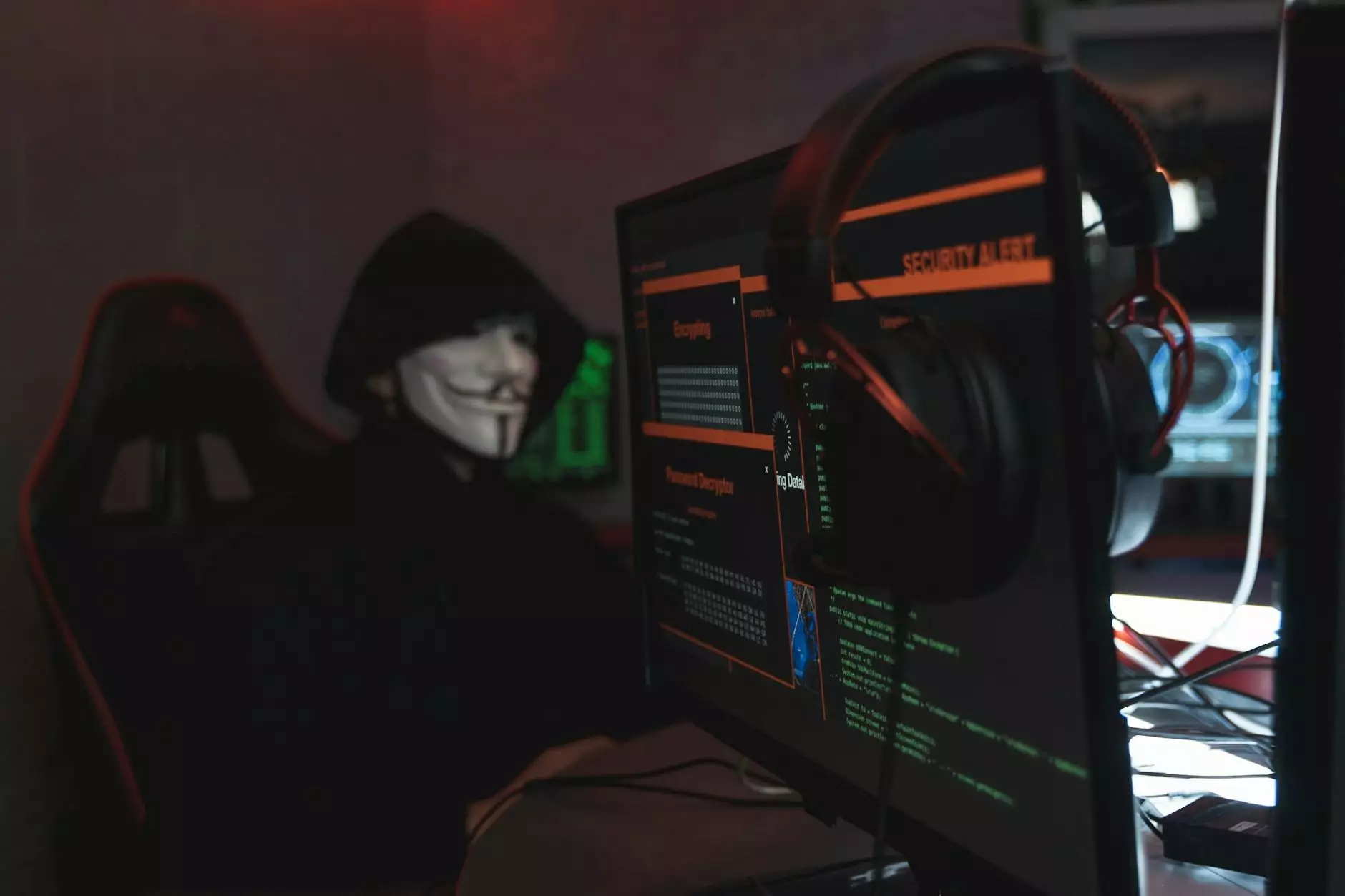
In the digital age, the threat of malware has become an omnipresent concern for businesses of all sizes. A significant advancement in combating these challenges is the integration of machine learning malware analysis. Organizations, particularly those in the realm of IT Services and Computer Repair, are increasingly relying on cutting-edge technologies to identify, understand, and combat malware threats effectively.
The Rise of Malware Threats
As technology evolves, so do the tactics and techniques employed by cybercriminals. Malware attacks have become more sophisticated, with cybercriminals continually finding new methods to infiltrate systems. In 2022 alone, it was reported that approximately 60% of all businesses experienced some form of cyberattack, leading to financial loss and damage to reputation.
Types of Malware and Their Impact
- Viruses: Malicious code that replicates itself within files.
- Worms: Self-replicating malware that spreads across networks.
- Trojans: Malicious software disguised as legitimate software.
- Ransomware: Malware that locks files until a ransom is paid.
Understanding Machine Learning in Cybersecurity
Machine learning (ML) is a subset of artificial intelligence (AI) that allows systems to learn from data and improve over time without being explicitly programmed. In the context of cybersecurity, ML algorithms analyze vast amounts of data to identify patterns and anomalies that may indicate a malware attack. This proactive approach is a game changer for businesses striving to enhance their security systems.
How Machine Learning Malware Analysis Works
Machine learning malware analysis employs various techniques to classify software as malicious or benign. The process typically involves:
- Data Collection: Gathering vast amounts of data on known malware and non-malware samples.
- Feature Extraction: Identifying key characteristics of the software that can indicate its behavior.
- Model Training: Using historical data to train ML models to recognize patterns.
- Real-Time Analysis: Deploying these models to monitor and analyze new files and behavior in real-time.
Benefits of Machine Learning Malware Analysis
1. Enhanced Detection Rates
Traditional signature-based detection methods are becoming increasingly ineffective due to the ever-evolving nature of malware. Machine learning provides dynamic detection capabilities that improve accuracy and reduce false positives. It can analyze files based on behavior rather than solely relying on known signatures.
2. Reduced Response Time
With the speed at which malware operates, time is a critical factor in mitigating threats. By employing machine learning, organizations can significantly reduce the time it takes to identify and respond to potential threats, ensuring that systems remain secure.
3. Cost-Effectiveness
Investing in advanced machine learning tools for malware analysis can lead to long-term savings. By preventing data breaches and system downtime, businesses can protect their bottom line while investing in robust security systems that provide lasting protection.
Implementing Machine Learning Malware Analysis in Businesses
To harness the power of machine learning malware analysis, organizations must consider the following steps:
1. Assess Current Security Posture
Before implementing new technologies, businesses should conduct a thorough assessment of their current security measures. This will help identify vulnerabilities and areas where machine learning can make a significant impact.
2. Choose the Right Tools
There are numerous machine learning tools available for malware analysis. Companies should select tools that are compatible with their existing systems and align with their specific security needs. Evaluating software based on scalability, user-friendliness, and technical support is crucial for successful implementation.
3. Employee Training
Implementing new technologies requires that employees are equipped to handle them. Providing thorough training on how machine learning malware analysis works and its benefits will empower employees to utilize these tools effectively.
4. Continuous Improvement
Cyber threats are constantly evolving, and so should the strategies to combat them. Regularly updating machine learning models and refining detection algorithms ensures that organizations stay ahead of emerging threats.
Challenges in Implementing Machine Learning for Malware Analysis
Despite the numerous advantages, integrating machine learning into malware analysis does pose challenges:
1. Data Privacy and Security Concerns
Organizations must ensure that the data used to train machine learning models adheres to privacy regulations such as GDPR and CCPA. Data security and compliance should be at the forefront of any machine learning initiative.
2. The Complexity of Implementation
Implementing machine learning solutions can be complex and time-consuming. Lack of in-house expertise can hinder progress, necessitating partnerships with specialized vendors or consultants.
3. Dependence on Quality Data
The effectiveness of machine learning in malware analysis largely depends on the quality of the input data. Insufficient or biased data can lead to inaccurate models, potentially compromising security measures.
Future Trends in Machine Learning Malware Analysis
1. Increased Use of Behavioral Analysis
As machine learning algorithms advance, there will be a greater focus on behavioral analysis tools that monitor user behavior and system interactions to identify potential threats based on unusual actions.
2. Integration with Other AI Technologies
The future of malware analysis will likely see machine learning being integrated with other artificial intelligence technologies, such as natural language processing (NLP) and automated response systems, enhancing overall security protocols.
3. Growth of Autonomous Security Systems
We can expect to see the rise of autonomous security systems that rely heavily on machine learning for real-time threat detection and mitigation, improving overall organizational resilience to cyber threats.
Conclusion
In conclusion, machine learning malware analysis represents a transformative approach in the ongoing battle against cyber threats. For businesses, especially those in the IT services sector, embracing these advanced analytical capabilities is not just an option; it is a necessity. By implementing comprehensive and intelligent solutions, businesses can enhance their security postures, respond faster to threats, and significantly reduce their risk of cyberattacks. As technology continues to evolve, the integration of machine learning into security strategies will be crucial for staying one step ahead of cybercriminals.
For more information on enhancing your business’s security with machine learning solutions, visit spambrella.com.